Impact of Artificial Intelligence over-reading for instant feedback on quality of home spirometry
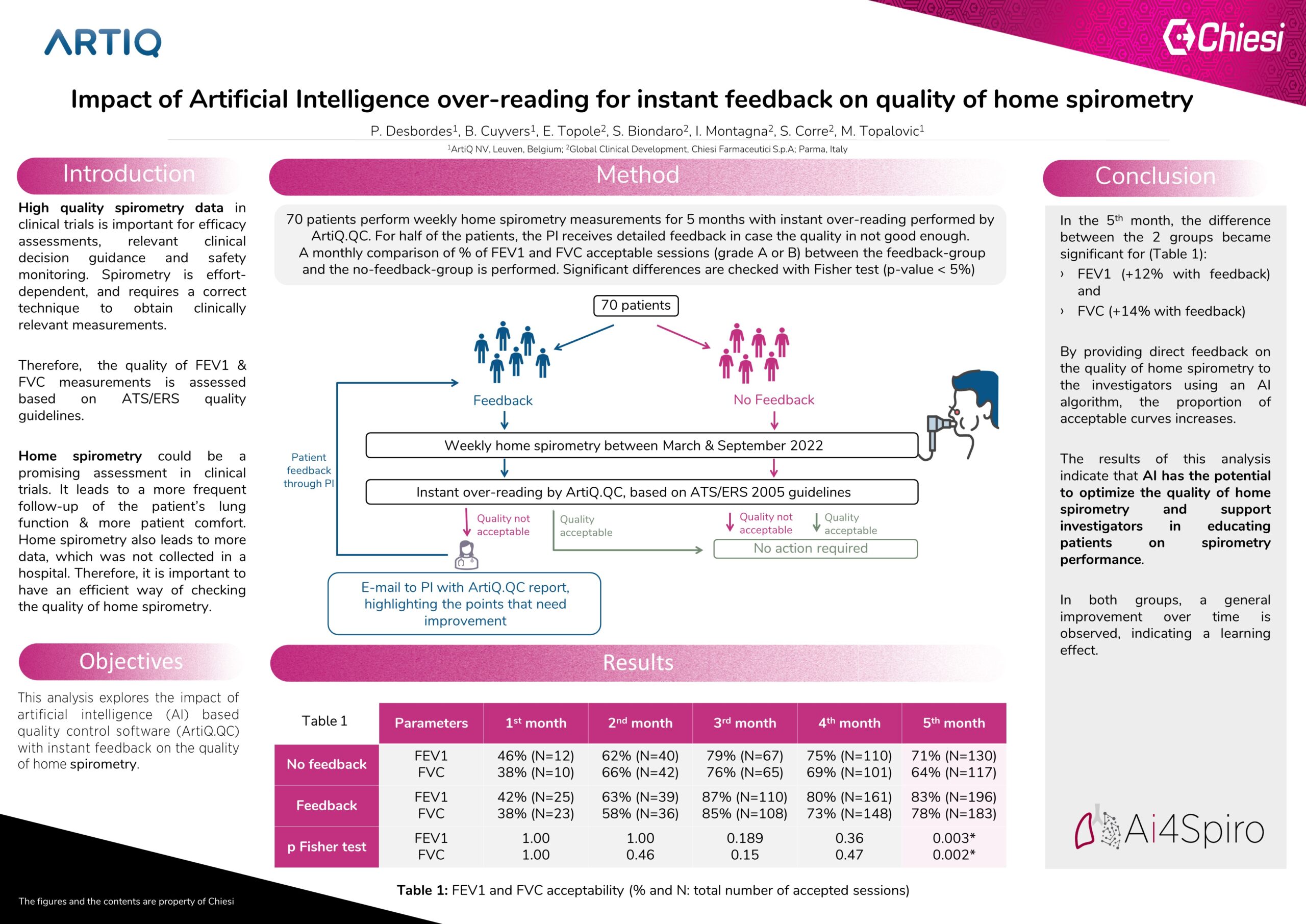
Rationale:
When performing spirometry, the acceptability of FEV1 and FVC are assessed based on the ATS/ERS quality guidelines. High quality of these parameters in clinical trials is important to assess efficacy, guide relevant clinical decisions and safety monitoring. Spirometry in the home setting is being explored as a promising assessment in many clinical trials. The amount of data generated is demanding to manually process in terms of quality assessment. In this study, we are investigating the impact of instant feedback provided by an AI algorithm (ArtiQ.QC) on the quality of home spirometry.
Methods:
Weekly home spirometry data of 70 patients enrolled in an asthma clinical trial, has been prospectively followed between March and September 2022 by the AI algorithm. AI-based software (ArtiQ.QC v1.3.0, ArtiQ NV, BE) is capable of an instant quality check of both qualitative and quantitative parameters. Each home spirometry session was assessed by the AI algorithm, for both FEV1 and FVC parameters. Acceptability is based on 2005 guidelines [1] due to the capability of the home spirometer. For half of the randomly picked subjects, AI-based feedback is provided to the investigators highlighting the points needing improvement. The second half was also AI-assessed, with no feedback provided. Each month, the proportion of FEV1 and FVC acceptability (defined as grade A or B) is assessed and compared between the two groups. A Fisher test is used to check for significant differences (p-value < 5%).
Results:
A general improvement over time in FEV1 and FVC acceptability is observed in both groups, indicating a strong learning effect (Table 1). The acceptability rate of FEV1 is slightly higher than the one of FVC. Improved acceptability for both FEV1 and FVC from the 3rd month onward in the group with instant feedback was observed. Difference between the groups was significant at month 5 (p = 0.003 for FEV1 and 0.002 for FVC). In the last period, the difference between the 2 groups was 12% for FEV1 and 14%for FVC.
Conclusions:
By providing direct feedback on the quality of home spirometry to the investigators using an AI algorithm, the proportion of acceptable curves increases. This indicates that AI can optimize the quality of home spirometry and support investigators in educating patients on spirometry performance.
Authors:
P. Desbordes1, Cuyvers1, E. Topole2, S. Biondaro2, I. Montagna2, S. Corre2, M. Topalovic1
Affiliations:
[1] ArtiQ NV, Leuven, Belgium
[2] Global Clinical Development, Chiesi Farmaceutici S.p.A; Parma, Italy